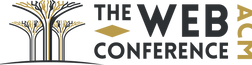
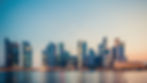
GFM 2024
The WebConf Workshop on Graph Foundation Models
May 13, Singapore
Overview
The AI landscape is witnessing a transformative shift with the advent of foundation models, like ChatGPT and GPT-4, which are pre-trained on broad data and can be adapted to a myriad of downstream tasks. They have showcased astounding reasoning capabilities, revolutionizing areas such as linguistics and vision.
However, the inherent complexities and pervasiveness of graph data, with its unique relational characteristics, present both challenges and intriguing opportunities for these foundation models. Given the extraordinary achievements of foundation models and the omnipresence of graph data, addressing this divergence becomes crucial.
Pivoting towards this emerging need, we introduce the "Graph Foundation Model Workshop" (GFM Workshop), envisioned as a pioneering foray into the intricate web of Graph Foundation Models (GFMs). Our workshop is meticulously crafted to mitigate the existing disparities between foundation models and graph machine learning, thereby fostering a convergence that can potentiate novel applications and theoretical advancements. Potential topics to be explored during the workshop include a thorough discussion of applications of existing foundation models to graphs, the design of foundation models for graphs, and a speculative glance toward future opportunities and applications. We are confident that our workshop will set the stage for a synergistic blend of graph and natural language processing, heralding new avenues in research and real-world applications.
Call for Papers
Submission website: https://easychair.org/conferences/?conf=thewebconf2024_workshops
Submission deadline: February 15, 2024
Submission site: https://easychair.org
Author notification: March 4, 2024
Camera-ready deadline: March 11, 2024
Workshop (in person): May 13, 2024 (Monday)
We welcome submissions regarding the foundation models for graphs, including but not limited to:
-
Graph-specific foundation models: Innovative ideas and perspectives in building generic foundation models for graph-structured data and relational data. Examples include scaling and extending graph-specific models like Graph neural networks to do pre-training and adapt to downstream tasks. Typical a special focus on the graph foundation model on big network (e.g., Google scale) and AI4Science application (e.g., computational biology, and chemistry).
-
Data-centric perspectives on graphs: Ideas and proofs-of-concept in solving graph-related problems from a data-centric perspective. Examples include how to enhance graph data availability and quality, and how to learn from graph data with limited availability and low quality.
We invite submissions of both short research papers, with a maximum of 4 pages (excluding references and supplementary materials), and full-length research papers, extending up to 8 pages (excluding references and supplementary materials). There are up to 2 additional pages for references and optional appendix. Each accepted paper will be presented in a poster format.
Submissions must adhere to the ACM template and formatting guidelines. It is not necessary for authors to include the checklist provided in the template. Papers should be submitted in .pdf format. Our review process is double-blind, and as such, submissions should be appropriately anonymized. We also accept papers that have been previously published or are currently under review.
Schedule
9:00-9:30
Giving a Voice to Your Graph: Data Representation in the LLM Age
Bryan Perozzi
10:30-11:00
Coffee break
N/A
12:00-13:30
Lunch Break
N/A
9:30-10:00
Multi-modal foundation AI models for molecules and drug design
Marinka Zitnik
11:00-11:30
Foundation Models for Knowledge Graph Reasoning
Michael Galkin
13:30-14:30
Panel Discussion
Xavier Bresson, Yuan Fang, Qian Liu, Michael Galkin, Zhen Li
10:00-10:30
Enhancing Foundation Models with Relational Data and Relational Reasoning
Rex Ying
11:30-12:00
Foundation Models for Text-Attributed Graphs
Bryan Hooi
14:30-15:00
Coffee break
N/A
Keynote Speaker
Panel Speaker
Panel Discussion
Panelist
Moderator

National University of Singapore

Zhejiang
University
Advisory Board

Mila - Quebec AI Institute

Amazon.com

University of Oxford

Amazon.com

National University of Singapore

National University of Singapore

Amazon.com

Michigan State University